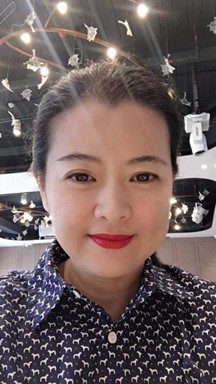
Massive amounts of data points are generated by transportation infrastructure, traffic, travel demand, autonomous and connected vehicles (CAVs), and intelligent transportation systems (ITS). This data must be processed and analyzed. The question is, how can all this data be captured and processed in an efficient way to solve transportation engineering’s most pressing issues?
That is what Di Wu, a doctoral student in transportation engineering at Jackson State University, is working on. She is exploring ways to automate the plethora of data generated by transportation infrastructure as it relates to CAVs and safety.
“With the development of ITS and connected and automated vehicles, the massive influx of data provokes the ever-increasing demand for data processing and analyzing,” Wu said. “It is imperative to apply the cutting age technology of Big Data analytics and AIoT (artificial intelligence of things) to transportation engineering to systematic automation, which is the goal of Big Data in transportation engineering.”
Under the guidance of her doctoral program advisers at Jackson State University, Dr. Robert Whalin and Dr. Shuang Z. Tu, Wu is working on two STRIDE projects which address the automation of transportation data: 1) Automatic Safety Diagnosis in a Connected Vehicle Environment (Project F4) and 2) Real-time Safety Diagnosis System for Connected Vehicles using Parallel Computing (Project O6).
The first project, which is almost completed, has generated a computational near-crash warning system, which uses basic safety message data generated from connected vehicles (CVs). The collision warning tools that currently exist on vehicles rely mostly on the data collected by the vehicle’s own sensors, such as camera, LIDAR, or radar, but the newly developed tool adds an extra layer of protection.
“This newly created tool can be used as an additional collision warning tool,” Wu said. “In the case of vehicle’s sensors are malfunctioning, the existing tools might shut down, but the newly created warning system can still keep functioning.”
Wu said that in the short-term, the impact generated by this research project would be in decreasing the number of traffic accidents, lives saved, and decreases in property damage. In the long-term, the impact would be to improve the safety of transportation automation.
The second project (Project O6), which was recently awarded, will implement parallel computing to the computational near-crash warning system. Parallel computing is essentially a higher end type of computing. It is a computation paradigm in which many calculations or processes are carried out simultaneously. It can speed up computational processing and problem solving.
In Project F4, sequential codes, the computational program that is designed for one computer thread, were used to computationally program the near-crash warning system. However, its capacity is limited because it processes the computations one by one. As more and more CVs flood the market in the near future, the computational system created in Project F4 will be too slow to complete the increased computation in time.
Wu says the goal of Project O6 is to upgrade the product generated as a result of Project F4 to a parallel version.
“It will adopt the state-of-art parallel computing techniques in the safety diagnosis system,” she said.
When Du is not working on her data-intensive research projects, she likes to practice Taiji (Tai Chi), which is a mind-body practice that originated in China as a form of martial art.